Smart mobility is one of the grand technological challenges of cyber-physical systems in the era of artificial intelligence and the Internet-of-Things. More specifically, autonomous-driving controllers are a major priority of our modern society with the great promise of dramatically reducing human fatalities and to decrease pollution. While many advances have already been achieved over the past decade, such as Tesla’s cars driving in Autopilot mode on various roads within the US, the very accidents of some of these cars clearly expose the vulnerabilities of the current state-of-the-art. In particular, highly automated vehicles appear to ignore some of accepted principles for the design of fault-tolerant systems; certification of object-recognition techniques is causing major concerns, and the psychology of pedestrians and car drivers in mixed-traffic together with autonomous cars is largely unexplored.
Will we ever be able to fully trust autonomous-driving controllers? What is needed so we can light-heartedly entrust our children to such highly automated vehicle, having them shuttled unattended across town?
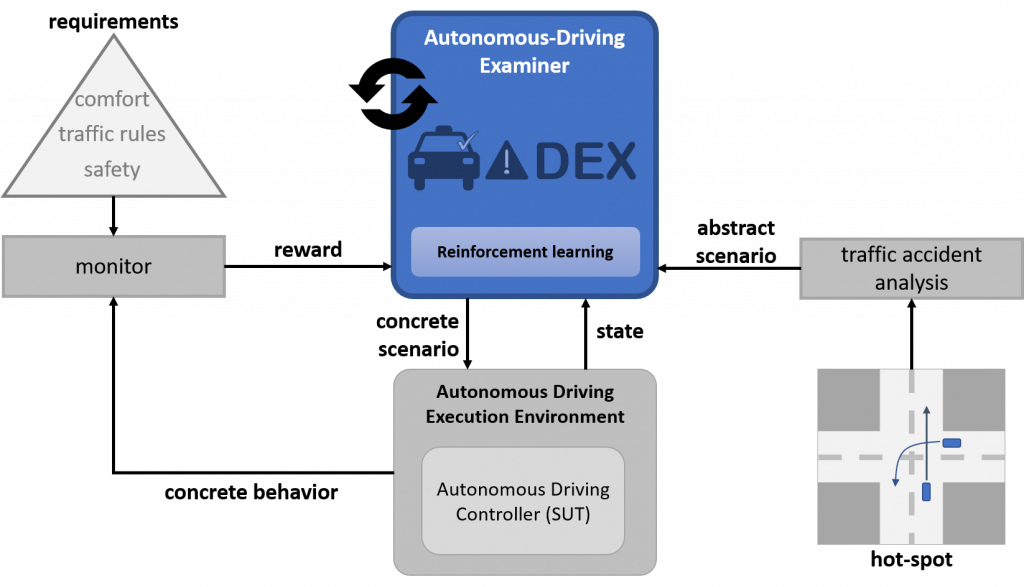
Human drivers have to prove their abilities to a driving examiner, before being allowed to drive autonomously. Following this analogy, we propose in ADEX a multidisciplinary approach to the design and synthesis of a comprehensive, human-centric, autonomous-driving examination, which is agnostic to the particular design (i.e., independent of its underlying concept) of an autonomous-driving controller, to expose its vulnerabilities or unknown flaws. The driving examination will consist of a large set of realistically simulated traffic situations, called scenarios, with human behavioral models for pedestrians and drivers, and various weather and road conditions. While the analysis and testing of the behavior of the autonomous-driving controller in normal traffic conditions will play a very important role, a special emphasis will be put on edge cases rarely occurring in normal traffic, to allow uncovering hidden issues. For the autonomous-driver such edge cases might represent system limiting situations. Analysis of real-world traffic accidents will be used to synthetically generate new, realistic and critical traffic scenarios for testing. Causes of accidents are often resulting from the interaction of technical and human (psychological and physiological) factors and both will be studied using holistic accident analysis on hot spots. Actions of the autonomous-driving controller will be thoroughly quantified in form of rewards, that will be exploited by reinforcement-learning techniques, to more and more generate traffic situations in which the autonomous-driving controller would fail (towards safety, regulation and comfort criteria). As for the human-driving examination, if an autonomous-driving controller passes it, we as a society will achieve a greater confidence in the system’s reliability and will be more inclined to accept it on our roads.
The proposed work is driven by an interdisciplinary consortium, with experts in artificial intelligence, safety, fault-tolerant design, cyber-physical systems, psychology and standardization efforts in autonomous driving. ADEX aims at developing a trustworthy framework for automatically examining autonomous-driving controllers.